Solving a formerly unsolvable drinking water challenge
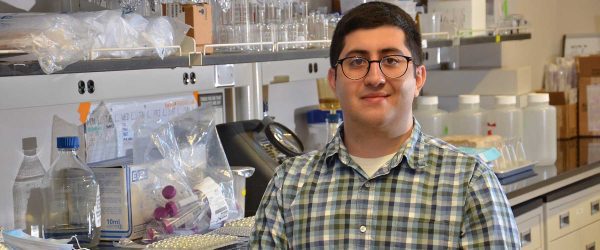
When Civil Engineering student Amir Alansari learned of a drinking water treatment challenge that no one had been able to solve for almost 50 years, he knew he had to give it a try. The challenge became an “obsession” as he reinvented scientific methodology, collected massive amounts of data and adapted new technology to analyze that data. The complexity of the project added years to his graduate studies at UNC Charlotte, but he has now succeeded where so many others have failed.
A Civil Engineering doctoral student since 2013, Alansari began his Lee College of Engineering career as an undergraduate in 2007, before completing a master’s degree.
“It has been a long road,” Alansari said. “I figured out that I’ve been here longer than 36 of the buildings on campus. What has kept me here so long has been a passion to scientifically optimizing drinking water treatment.”
Originally from Dubai, Alansari became interested in drinking water during his junior year, when he did some undergraduate research on desalinization. He expanded his research scope to more complex topics of water treatment during his graduate studies.
“I like drinking water research, because it involves so many disciplines, including engineering, biology and chemistry,” Alansari said. “The more I study it, though, the more I realize how much I don’t know. But it is an essential problem for the entire planet and for me the research is fascinating.”
Alansari’s doctoral research focuses on how to predict and optimize drinking water treatment in order to produce cleaner water more efficiently. Because of the huge number of variables involved in water treatment, it is a research challenge that has baffled scientists and engineers for decades.
James Amburgey, associate professor of Civil Engineering and Alansari’s graduate mentor for more than a decade, told a story about how the legends of early water-quality research couldn’t determine what variables impacted water treatment in what ways.
Alansari said, “I wanted to know why they couldn’t. It became an obsession for me. Dr. Amburgey allowed me to start my research on the topic, but said I would have to start from scratch and ignore what everyone else had done. He wouldn’t even let me read the old research reports, so I wouldn’t be influenced by them.”
Two of the main components of water treatment are sedimentation, where water is kept still and particles are allowed to settle to the bottom, and filtration, where water is run through filter media (such as sand) to remove particles. The problem is that most particles in water are too small to settle or filter on their own. If particles can be made to stick together and become larger, they can be more effectively removed through the two processes.
Getting the particles to stick together is known as coagulation, which is achieved by adding chemicals (coagulants) to water. Coagulation is the first and most important step of drinking water treatment, because the efficiency of every subsequent process is directly dependent on the efficiency of coagulation.
The great difficulty with optimizing coagulation for drinking water is there are at least 15 variables that impact the performance of the process. The variables include everything from water chemistry, to weather, to pH levels, to the individual characteristics of each treatment plant, and all of these variables change constantly.
“Most of the coagulation studies done in the past were either site-specific or focused on one variable, and hence did not apply to most real-world conditions,” Alansari said. “Developing a universal and practical model of coagulation has been a near-impossible task, because of the chemical complexity of water and the sheer number of factors and their interactions that determine the performance of the coagulant.”
To succeed where others had failed, Alansari first designed and built a jar-test apparatus that better mimics water-treatment plants by including sedimentation and filtration stages. The apparatus has six side-by-side jar sections, which allows variables to be adjusted in each and results compared immediately.
“I had to kind of reinvent how we do jar testing,” Alansari said. “A traditional jar test didn’t include a filtration stage, and if you base results off just settling, you can’t determine the most-effective chemical treatment. My setup represents everything that goes on in a treatment plant.”
Using the new jar-test method, Alansari concentrated on analyzing six of the primary water-treatment variables. This research of varying one factor at a time in side-by-side tests created massive amounts of data. The second phase of Alansari’s research was the even more complex task of turning the data into usable information for determining coagulation optimization.
“Where Amir shines is when he comes up against a project that is almost impossible to solve,” said Amburgey. “And Amir is a little modest. He took on a project that had never been solved, which is to model coagulation. It is a very complicated process, and prior to Amir nobody could figure it out.”
Along with building the jar-test system, another key element in Alansari’s research was to make his own synthetic (manmade) water. By creating 16 different types of synthetic water, Alansari was able to mimic streams, lakes and other water systems. The synthetic water was made by starting with ultrapure water and then adding contaminants and adjusting pH levels. The synthetic water allowed Alansari to precisely control variables, as opposed to using samples taken from actual lakes and streams.
“Amir has done some incredible things,” Amburgey said. “He took a jar-test method that didn’t work and made it work. He created his own method, he created his own water, and now by using machine learning, he is creating his own computer model. No one has been able to do this before.”
The final stage of Alansari’s research is to make the information useful to drinking water treatment plant operators. This means converting the huge amounts of data into usable information for determining the best methods for coagulation. To achieve this, Alansari is employing machine-learning techniques. A subset of artificial intelligence, machine learning is the use of computer algorithms that improve automatically through experience.
“Applying machine learning is helping me develop a universal model for coagulation,” Alansari said. “The model takes all the data I collected and tries to ‘learn’ complex hidden patterns, which we know are there but don’t quite understand. What used to take me about two weeks to produce in the lab now can be done in a matter of seconds since the model learns how to perform virtual jar tests for practically any water.”
With the help of machine learning, Alansari is developing a computer modeling application that will take guess work out of treating water. The tool will allow treatment plant operators to determine optimal treatment methods and know why what they did had what result.
“I’m working now on a better interface to make the model more user-friendly,” Alansari said, “The interface will make it easier for water plant operators to use.”
Alansari has developed a beta version computer application of his jar test systems known as the JT Wizard, which is available on his research website. To date, more than 150 people have downloaded the Wizard.
Presentations of Alansari’s work have been well received. In 2019, he won the regional and national Fresh Ideas Poster Competitions at the American Water Works Association’s (AWWA) annual conference and exposition for “Next-Generation Coagulation Optimization for Drinking Water Treatment Plants: A Case Study.” The National Society of Professional Engineers named him its Milton F. Lunch Ethics Contest winner in 2018. He also won a best paper award from AWWA in 2016 for “Optimization of Coagulation Pretreatment Conditions in Ceramic Membrane System.”
Alansari said, “The research started as an obsession to try and succeed where others could not. As the project has gone on, I have gotten even more passionate about the research. I applied new methods and technology to the theories of the ’50s and ’60s, and they have been successful. To me, it’s been very fulfilling and fun.”